https://www.gislounge.com/using-cell-phone-data-map-
urban-activity-patterns/
MAY 15, 2018BY MARK ALTAWEELSPATIAL ANALYSIS
With the widespread presence of mobile telephones phone providers now collect location data throughout the day via GPS data and cellular signals. This opens up both spatial research opportunities but also concern in data protection.
Methods have been developed also to counteract the sometimes patchy or low resolution location data obtained. Such data can make it difficult to create accurate reconstruction of where and when people travel to given locations. Algoriths to filter and smooth data allow interpolated location and time to be determined, where trajectories can be used to estimate direction and given speed at a location used to estimate overall pace of travel. Once the data is cleaned, using Markov networks, or probabilistic understanding of network options and choices, routes to activities and traffic to events could be mapped and estimated for groups of data. This allows a relatively accurate understanding of activity clusters as well as the modeling and forecasting of potential activities or even where people might go once an activity has concluded.[2]
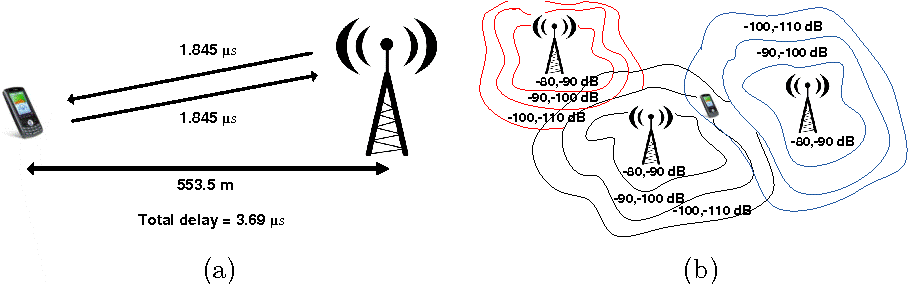
METHODS OF ESTIMATING MOBILE PHONE LOCATION INFORMATION: (A) TIME ADVANCE AND (B) RECEIVED SIGNAL STRENGTH TECHNIQUES. IMAGE: CALABRESE FERRARI, & BLONDEL, 2014.
Because data from cellphones are often coarse and generalized to anonymize it, segmenting the data into activity zones can be a challenge for researchers. One method is to compare activity by dividing an urban zone into districts of activities. This means measuring baseline activity on normal days, while then measuring what activities occur during other, non-normal days across zones to detect anomalies from cellular data. By detecting anomalies, and mapping those to events or other known activities in the city, one can begin to determine how economic, social, cultural and other events affect and relate to the wider urban population. In other words, segmentation and detecting when cellular trends differ from the baseline enable a measurement of given events’ effects on the population and how attractive those events might be.[3]
Aggregate flows between regions could also allow an understanding of daily mobility patterns or even the effect of events on mobility patterns across larger scales. For instance, research was able to show how population patterns and flow between Paris and surrounding regions developed over the course of time or because of key transport nodes.[4] Other potential use of large-scale mobile phone data is planning for evacuation. Mobile phone data does enable an estimate of how people evacuate in a major incident. For instance, during a major incident, people often attempt to reuse a given transport network even after it has been severely affected. This could potentially impede rescue work that is ongoing and often may mean first responders have to plan activities around resumption of services in transport networks that provide fewer redundant options.[5]
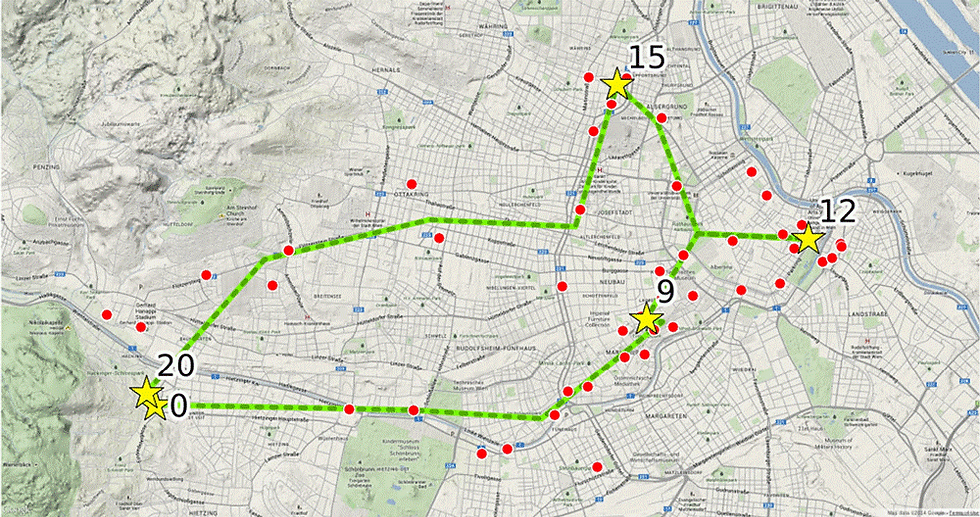
RECONSTRUCTION OF TRIPS AND VISITED PLACES. RED DOTS REPRESENT THE RAW CELL LOCATIONS AND THE GREEN LINE IS THE FILTERED TRAJECTORY. YELLOW STARS MARK VISITED PLACES AND THE NUMBERS INDICATE THE TIME OF DAY IN HOURS SINCE MIDNIGHT. SOURCE: WIDHALM ET AL., 2015.
Mobile cellular data has a major potential in allowing a better understanding of how we use urban spaces, including transport networks and access to events and venues. It also offers potential in understanding our economic activities through comparisons between normal and anomolous traffic patterns. The danger, however, is that such data could be potentially stolen. While current laws generally do attempt to protect the individual, the danger still exists for individuals to be determined by possibly stored location data that is subsequently stolen.
References
[1] For a survey on regulations and general usage of cellular data, see: Calabrese, F., Ferrari, L., & Blondel, V. D. (2014). Urban Sensing Using Mobile Phone Network Data: A Survey of Research. ACM Computing Surveys, 47(2), 1–20. https://doi.org/10.1145/2655691.
[2] For more on how errors and low resolution in cellular data could be overcome, see: Widhalm, P., Yang, Y., Ulm, M., Athavale, S., & González, M. C. (2015). Discovering urban activity patterns in cell phone data. Transportation, 42(4), 597–623. https://doi.org/10.1007/s11116-015-9598-x.
[3] For more on segmentation and detection of anomalies in urban activities, see: Cici, B., Gjoka, M., Markopoulou, A., & Butts, C. T. (2015). On the Decomposition of Cell Phone Activity Patterns and their Connection with Urban Ecology (pp. 317–326). ACM Press. https://doi.org/10.1145/2746285.2746292.
[4] For more on urban-rural and nationwide population flows between cities and countryside, see: Trasarti, R., Olteanu-Raimond, A.-M., Nanni, M., Couronné, T., Furletti, B., Giannotti, F., … Ziemlicki, C. (2015). Discovering urban and country dynamics from mobile phone data with spatial correlation patterns. Telecommunications Policy, 39(3–4), 347–362. https://doi.org/10.1016/j.telpol.2013.12.002.
[5] For more on and an example of mass incidents and response and evacuation using mobile phone data, see: Duan, Z., Lei, Z., Zhang, M., Li, W., Fang, J., & Li, J. (2017). Understanding evacuation and impact of a metro collision on ridership using large-scale mobile phone data. IET Intelligent Transport Systems, 11(8), 511–520. https://doi.org/10.1049/iet-its.2016.0112.